Artificial intelligence (AI) and machine learning are at the centre of innovation in 2020. Businesses and governments have and are continuing to invest heavily in the development and implementation of AI across the technology, finance, automotive, pharmaceuticals, and consumer insights industries. AI-led technology has already become a key driver in significant profit centres, benefiting those who succeed with a monetisable information advantage.
However, development of any market-leading advantage should be done with an awareness of how to protect that investment and retain the advantage. So, can you get a monopoly on your AI's lucrative invention? This article considers potential hurdles to patent protection and some suggested solutions.
The IP frameworks are already being tested with respect to AI inventions. At the intergovernmental level, WIPO, on December 13 2019, published an issues paper in calling for submissions on the interaction between AI and IP. At the industry and academic level, the application to the EPO in August 2019 (rejected on December 20) for patents in the name of DABUS, an AI tool relying on a system of deep neural networks.
Much of the industry and academic (and now patent office) focus has been on whether the patent framework is capable of allowing for an inventor which is not a legal person. Currently, the answer from the EPO, the USPTO, the UKIPO and others is no, but it seems that at some stage, inventorship, or a path to ownership at least, could potentially be solved by granting of limited legal personhood to AI if the policy arguments in favour of AI inventions win out.
However, there is perhaps a more intractable problem. Regardless of the jurisdiction, the fundamental patent bargain is that the inventor obtains a monopoly in return for disclosing the invention and dedicating it to the public for use after the monopoly has expired. While the precise wording differs jurisdiction by jurisdiction, it is a requirement for validity that a patent application discloses the invention in a manner which is "sufficiently clear and complete for it to be carried out by a person skilled in the art". Inventions created by AI harbour particular issues on a practical level for this sufficiency requirement.
Is there an inherent problem of sufficiency for any invention created by AI?
Many of the current advancements produced by AI are created by 'black box' AI. Humans may understand the input and output of the AI tool but the process of the invention may remain hidden in the sense that its developers are not always able to understand what the AI is doing because of the computational and dimensional power of the AI, explained further below. Of course, we do know that data holds insights that can help enable better decisions or inventions, and the aim is to process the data in a way that extracts insights and enables users to take action. Humans and AI are both processors but with different abilities. AI uses advances in processing power, data storage capability and algorithmic design to perform tasks in fundamentally different ways to human beings.
As a result, AI often relies on machine-learning algorithms that deal with data in ways that are not easily understood by humans – that is, the mechanics, or enablement, of the invention itself cannot be properly disclosed as there is insufficient understanding of how the invention is performed. For example, AI systems used to predict embryo quality for selecting human blastocysts (the precursors to embryos) for transfer in IVF are being fed images we understand and provide an output we understand (suitability) but offer no insight on why its predictions are better than those of embryologists. This AI is a deep neural network – that is, it uses thousands of artificial neurons to learn from and process data. The complexity of these neurons and their interconnections makes it very difficult to determine and explain how decisions are being made.
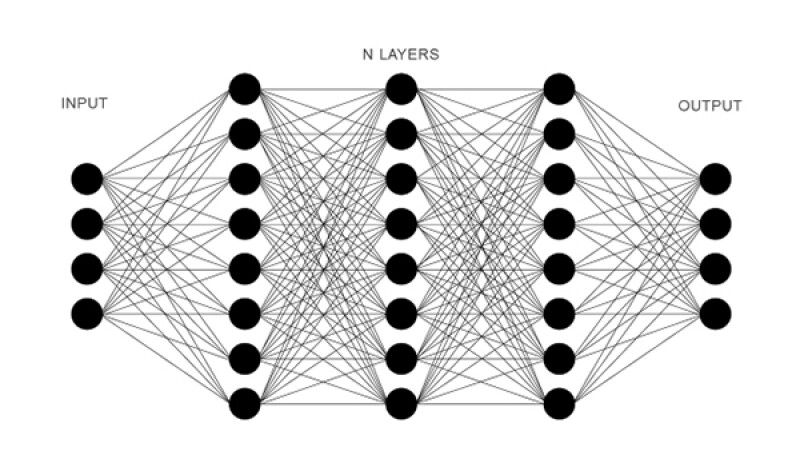
Other sophisticated forms of AI, for example support-vector machine-based AI, can have the same issue because they process and optimise numerous variables at once in higher dimensional spaces. This prevents humans from conceptualising how the AI tool is making decisions. One of the key benefits of AI is that it can process data and information in way that humans cannot.
This is excellent for the advancement and expansion of research, and application beyond the limitations of human computational power, but leaves us with a problem of the patent bargain – what can the humans involved with an AI invention do to ensure sufficient disclosure to the public (and therefore obtain a monopoly), particularly where the inventor may be non-human?
Can an AI invention be 'sufficiently clear and complete'?
If the AI system is developed without consideration to how an invention might be disclosed, it is unlikely it will be able to satisfy the requirement for a sufficiently clear and complete disclosure. We focus here on the problem of explainable AI, but we note that the ability to interpret AI also relies on transparency, i.e. the structural or coding details for the initial model.
We address two potential ways to deal with the disclosure hurdle.
(i) Ability to interpret explainable AI
The most powerful option is to actively push the research direction to develop AI in a manner that makes sure the AI tool is capable of explaining itself.
This entails significant cost and engineering challenges – explainable AI is an expensive and difficult area. Explanation needs to be built in up front. Unlike with humans, AI cannot readily explain things after the fact unless it has been programmed to do so in advance. At the moment, building in this type of explanation involves a trade-off of accuracy and sophistication. Currently, explainable systems require a model interpreter – which maps concepts onto the collection of features. So, a model could explain to you that it has identified a dog because it has identified paws, fur, a tail, etc., but this is not achieving anything more than a human could do (even if it can do it faster). We know already from use of AI in diagnostics for embryo candidates and identifying cancerous cells that the benefits of AI are not just for doing the same thing faster, but doing something differently, and more accurately and efficiently than human experts can, or doing something entirely novel.
In the AI sphere a more complex model will generally be more accurate but less easy to interpret. Explainable AI is an active area of research, but not yet necessarily a focus of all companies who in the future may wish to patent their AI. This research is being led in industries such as health and defence – for them the development of AI that explains its predictions or processes is critical from a legal, social and reputational standpoint. DARPA, the US's Defence Advanced Research Projects Agency, is a leader in explainable AI research, noting that "explainable machine learning will be essential if future warfighters are to understand, appropriately trust, and effectively manage an emerging generation of artificially intelligent machine partners."
The case of researching (and obtaining a commercial advantage with respect to) explainable AI is also relevant when applying AI to non-inventive scenarios. The EU General Data Protection Regulation introduces a right of explanation for individuals to obtain "meaningful information of the logic involved" when automated decision-making takes place with "legal or similarly relevant effects" on individuals. Without an explainable AI system, this may in effect outlaw many AI decision-making systems. A similar problem arose in a 2018 taxation case in Australia. The Full Federal Court found that under the relevant Tax Act, in order for there to be a "decision" by the Australian Taxation Office under the statute, "there must be a mental process of reaching a conclusion and an objective manifestation of that conclusion." In this case, a letter created by "deliberate interactions between [an officer of the Australian Taxation Office] and an automated system designed to produce, print and send letters to taxpayers" was not binding. These examples show the tip of the commercial advantage in developing explainable complex AI.
Given the complexity and trade-offs explained above, the development of explainable AI as a way of overcoming the sufficiency problem is only relevant for companies investing significantly in AI-driven solutions – where AI-led inventions will be at the core of their business. In those cases, a focus on explainable AI (which may be inventive in its own right) could hold a key to future patent protection for AI-led inventions.
(ii) Rely on the product
Another possibility to overcome the sufficiency hurdle is output specific – that is, it depends what the AI happens to create. For product claims, patent offices do not require humans to explain how they arrive at a conclusion, only to enable at least one way of performing the invention across the scope of the claim. A useful example is the case of DABUS, which is an AI tool developed by Stephen Thaler at the University of Surrey and has a "swarm of many disconnected neural nets" which combine and detach. DABUS's process led to the generation of two fractal-related inventions: patent applications entitled (1) "Food container" and (2) "Devices and methods for attracting enhanced attention".
Taking these examples, the descriptions for these patent applications look like any other; they are drafted by patent attorneys who have sought to distil the nature of the invention into a description, drawings and claims. It is less clear what explanation from the inventor, i.e. DABUS (or perhaps DABUS's creator Thaler), the patent attorneys had in order to understand the invention adequately to reduce it to writing. Any AI tool or its human team will need to be able to explain, at least to the patent attorney, what it has invented in order to obtain patent protection. Sufficiency for a single product requires at least one way of performing the invention, so if the process to make the claimed product developed by the AI system is common general knowledge (e.g. where the invention is a novel and inventive food container but, if shown to a packaging engineer, the container could be made based on known technology), it may be possible to meet the sufficiency requirement. It is therefore possible that, for some AI-led inventions, the invention or output itself will be clear enough to be disclosed in a manner enabling it to be performed, without understanding how the invention was developed, subject to the point addressed below about a 'person skilled in the art'.
How do we identify the person skilled in the art?
A more challenging question to resolve is for whom the disclosure must be sufficiently clear and complete – who is the person skilled in the art?
It could be, for example, a skilled AI tool or a person skilled in the field of the invention itself, or a skilled team which may encompass both.
If the skilled person is a skilled (non-inventive) AI system, then presumably one could test the sufficiency of the patent by feeding the patent's description and drawings as a piece of data to that AI tool and test whether it could follow the instructions and perform the claimed invention.
The first issue with this approach from a patent law perspective is how does this AI tool know what the skilled person (AI) could do with the patent at the priority date in light of the common general knowledge, and how could the AI system explain it (for example, in an expert report were the patent to be challenged)? If we are unable to have meaningful expert evidence about the state of the knowledge of the skilled person then it becomes very difficult to rely on the common general knowledge to support the sufficiency of the patent's disclosure, particularly where the definition of the common general knowledge is contentious.
|
Anyone developing an AI invention should ensure there is a framework to rely on trade secrets protection for the invention before considering patent protection |
|
Also, it may be difficult to accurately replicate an AI program as it was precisely at the priority date. In patent law, we currently rely on human experts to cast their minds back to what was common general knowledge at the priority date, but given the difficulties with retrospective explanation discussed above, this would appear to be very difficult (if not impossible in some cases) for AI. Further, at present, post-priority date experiments are extremely rare (if allowed at all) as persuasive evidence of priority date sufficiency or plausibility, so the idea of 'feeding data' to AI to test the sufficiency of the patent at the priority date would be contrary to that current way of thinking.
Second, and more fundamental, if the disclosure is only "sufficiently clear and complete" to an AI tool, not a human, there is a very real question of whether the patent has been made available to the public in a true sense. The patent bargain is not fulfilled if humans cannot take the benefit of the invention.
On the other hand, if the skilled person is a person, say a product engineer considering new food containers, there is a stark disconnect between the computing power and analytical ability of the AI inventor and how we judge the common general knowledge of the skilled person. Explainable AI would in principle be a necessary condition to compare between the AI inventor and human skilled person. Further, this conception of the human skilled person also creates issues regarding inventive step: what is inventive for our human skilled person may be trivial for an AI tool because of its computational power. But such an inventive step may also be unexplainable for anything other than AI. This in turn raises complex theoretical questions of whether the skilled person for the purposes of inventive step and sufficiency can readily be the same (which of course is a fundamental principle of patent law) in the AI context to ensure that AI patents do not fall at one (or both) of the inventive step and sufficiency requirements.
So, the identification of the appropriate skilled person and how that in turn affects the inventive step and sufficiency requirements will be one of the most legally complex issues for the patentability of AI-led inventions.
What should you do to protect your AI and its inventions?
Critical in all of this is some understanding and consideration at the outset of the type of invention you are aiming for – both in the short and long term. These should be considered in light of existing technical limitations of AI but with an awareness of moving beyond those.
Anyone developing an AI invention should ensure there is a framework to rely on trade secrets protection for the invention before considering patent protection. This should be presumed as the default. For a substantial part of an AI-led invention, for example where AI is used in high volume decision-making applications (think high frequency trading and online marketing), an accurate but hidden algorithm may be the commercially optimal approach.
However, those relying on or even contemplating use of AI-led inventions for big ticket advances, or platform technology development, should consider critically at the outset investing in concurrent development of making their AI explainable. Both the explainable AI itself and the potential for monopoly protection over its inventions present a real commercial opportunity, and explainable AI is one fundamental way to attempt to future-proof a company's R&D-based AI advancements.
![]() |
![]() |
Steven Baldwin |
Gabriella Bornstein |
Steven Baldwin is a partner, and Gabriella Bornstein an associate, at Kirkland & Ellis in London.